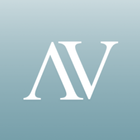
Description: Algovest is an AI-powered robo-advisor and trading platform that uses machine learning algorithms to optimize portfolios and automate investments. It aims to provide accessible, low-cost wealth management for retail investors.
Type: Open Source Test Automation Framework
Founded: 2011
Primary Use: Mobile app testing automation
Supported Platforms: iOS, Android, Windows

Description: Algoriz is an open-source data science platform that allows users to build machine learning models with no coding required. It has a visual interface for data preparation, model building, evaluation, and deployment.
Type: Cloud-based Test Automation Platform
Founded: 2015
Primary Use: Web, mobile, and API testing
Supported Platforms: Web, iOS, Android, API