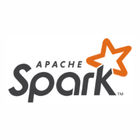
Description: Apache Spark is an open-source distributed general-purpose cluster-computing framework. It provides high-performance data processing and analytics engine for large-scale data processing across clustered computers.
Type: Open Source Test Automation Framework
Founded: 2011
Primary Use: Mobile app testing automation
Supported Platforms: iOS, Android, Windows
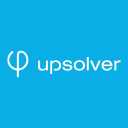
Description: Upsolver is a no-code platform for building and operating streaming data pipelines and analytics. It allows you to easily ingest, process, analyze, and visualize streaming data in real-time without managing infrastructure.
Type: Cloud-based Test Automation Platform
Founded: 2015
Primary Use: Web, mobile, and API testing
Supported Platforms: Web, iOS, Android, API