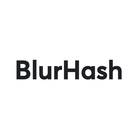
Description: BlurHash is an algorithm that creates a hash representation of an image which allows previewing that image as it loads, while using very little bandwidth. The hash is typically around 20-30 characters long.
Type: Open Source Test Automation Framework
Founded: 2011
Primary Use: Mobile app testing automation
Supported Platforms: iOS, Android, Windows
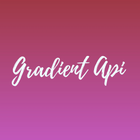
Description: Gradient API is an open-source tool for deploying and managing machine learning models. It allows data scientists to easily monitor, scale, and serve models in production
Type: Cloud-based Test Automation Platform
Founded: 2015
Primary Use: Web, mobile, and API testing
Supported Platforms: Web, iOS, Android, API