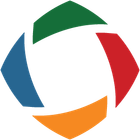
Description: CrossBrowserTesting is a cloud-based web application testing tool that allows users to test their websites and web apps across various browsers, operating systems and devices. It aims to provide comprehensive cross-browser compatibility testing.
Type: Open Source Test Automation Framework
Founded: 2011
Primary Use: Mobile app testing automation
Supported Platforms: iOS, Android, Windows

Description: Nerrvana is an open-source platform for deep learning research and development. It provides GPU-accelerated libraries, models, and tools for designing, training, and deploying deep neural networks.
Type: Cloud-based Test Automation Platform
Founded: 2015
Primary Use: Web, mobile, and API testing
Supported Platforms: Web, iOS, Android, API