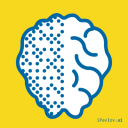
Description: DeepPavlov is an open-source library for building conversational AI assistants. It provides pre-trained models and tools for natural language understanding, question answering, document ranking and more.
Type: Open Source Test Automation Framework
Founded: 2011
Primary Use: Mobile app testing automation
Supported Platforms: iOS, Android, Windows
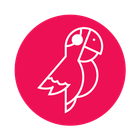
Description: ParlAI is an open-source software platform for developing conversational AI agents. It provides an interface to interact with different dialogue datasets, evaluate models, train new models from scratch, and integrate new datasets.
Type: Cloud-based Test Automation Platform
Founded: 2015
Primary Use: Web, mobile, and API testing
Supported Platforms: Web, iOS, Android, API