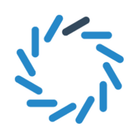
Description: Domino Data Lab is a collaborative data science platform that enables data science teams to develop, deploy, and monitor analytical models in a centralized workspace. It offers tools for model building, deployment, monitoring, and more with integrated security and governance features.
Type: Open Source Test Automation Framework
Founded: 2011
Primary Use: Mobile app testing automation
Supported Platforms: iOS, Android, Windows

Description: Cloudera CDH (Cloudera Distribution Including Apache Hadoop) is an open source data platform that combines Hadoop ecosystem components like HDFS, YARN, Spark, Hive, HBase, Impala, Kudu, and more into a single managed platform.
Type: Cloud-based Test Automation Platform
Founded: 2015
Primary Use: Web, mobile, and API testing
Supported Platforms: Web, iOS, Android, API