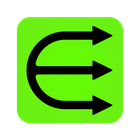
Description: Easy Data Transform is a desktop application for Windows, Mac and Linux that allows users to easily transform, clean, combine and manipulate data files in various formats like CSV, JSON, XML, databases and Excel. It has an intuitive drag-and-drop interface for transforming data between sources and destinations.
Type: Open Source Test Automation Framework
Founded: 2011
Primary Use: Mobile app testing automation
Supported Platforms: iOS, Android, Windows
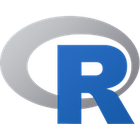
Description: R is a free, open-source programming language and software environment for statistical analysis, data visualization, and scientific computing. It is widely used by statisticians, data miners, data analysts, and data scientists for developing statistical software and data analysis.
Type: Cloud-based Test Automation Platform
Founded: 2015
Primary Use: Web, mobile, and API testing
Supported Platforms: Web, iOS, Android, API