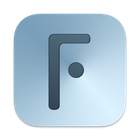
Description: Faraday.dev is an open-source collaborative platform for electrical and electronics engineers to share schematics, PCB layouts, code, and project documentation. It aims to improve engineer productivity and promote open hardware.
Type: Open Source Test Automation Framework
Founded: 2011
Primary Use: Mobile app testing automation
Supported Platforms: iOS, Android, Windows
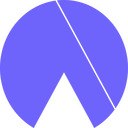
Description: Hypotenuse AI is an artificial intelligence platform that allows users to build customized AI solutions. It features drag-and-drop components to assemble AI building blocks, MLOps to deploy and monitor models, and support for all major machine learning frameworks.
Type: Cloud-based Test Automation Platform
Founded: 2015
Primary Use: Web, mobile, and API testing
Supported Platforms: Web, iOS, Android, API