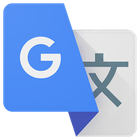
Description: Google Translate is a free translation service developed by Google that allows users to translate text, documents, speech, and websites between over 100 languages. It uses advanced machine learning and neural network algorithms to provide fast and accurate translations.
Type: Open Source Test Automation Framework
Founded: 2011
Primary Use: Mobile app testing automation
Supported Platforms: iOS, Android, Windows
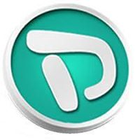
Description: Dilmanc is an open-source automatic differentiation software library for C and C++ programs. It allows users to numerically evaluate derivatives of C/C++ functions for applications such as gradient-based optimization and sensitivity analysis, without needing to derive and implement analytical derivatives.
Type: Cloud-based Test Automation Platform
Founded: 2015
Primary Use: Web, mobile, and API testing
Supported Platforms: Web, iOS, Android, API