
Description: H2O.ai is an open source AI and machine learning platform that allows users to build machine learning models for various applications such as predictive modeling, pattern mining, lead scoring, and fraud detection. It provides automatic data preparation, feature engineering, model building, model validation and model deployment.
Type: Open Source Test Automation Framework
Founded: 2011
Primary Use: Mobile app testing automation
Supported Platforms: iOS, Android, Windows
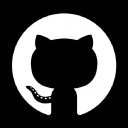
Description: Auto-sklearn is an open source machine learning library for Python that automates hyperparameter tuning and model selection. It builds on top of scikit-learn and uses Bayesian optimization to find good machine learning pipelines for a given dataset with little manual effort.
Type: Cloud-based Test Automation Platform
Founded: 2015
Primary Use: Web, mobile, and API testing
Supported Platforms: Web, iOS, Android, API