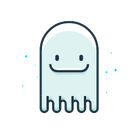
Description: Instadio is a software tool that helps organizations build and manage an internal podcast network. It provides an easy way to record, edit, publish, and analyze podcasts within a company.
Type: Open Source Test Automation Framework
Founded: 2011
Primary Use: Mobile app testing automation
Supported Platforms: iOS, Android, Windows
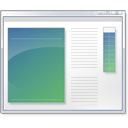
Description: Ras2Vec is a deep learning model for representation learning of cancer mutations. It encodes mutations into vector representations that capture similarities between mutations based on their proximity in protein structures. This enables better prediction of cancer driver mutations.
Type: Cloud-based Test Automation Platform
Founded: 2015
Primary Use: Web, mobile, and API testing
Supported Platforms: Web, iOS, Android, API