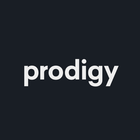
Description: Prodigy ML is an annotation tool that helps train machine learning models faster. It allows users to rapidly label datasets and build accurate models with less data.
Type: Open Source Test Automation Framework
Founded: 2011
Primary Use: Mobile app testing automation
Supported Platforms: iOS, Android, Windows
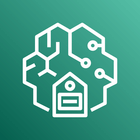
Description: Amazon SageMaker Data Labeling is a service that makes it easy to label your datasets for machine learning. You can request human labelers from a pre-qualified workforce and manage them at scale.
Type: Cloud-based Test Automation Platform
Founded: 2015
Primary Use: Web, mobile, and API testing
Supported Platforms: Web, iOS, Android, API