
Description: PROMT Translator is a powerful machine translation software that allows fast and accurate translation between over 50 languages. It features AI-powered translations, customizable dictionaries, and support for documents, websites, apps, and more.
Type: Open Source Test Automation Framework
Founded: 2011
Primary Use: Mobile app testing automation
Supported Platforms: iOS, Android, Windows
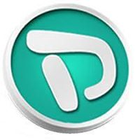
Description: Dilmanc is an open-source automatic differentiation software library for C and C++ programs. It allows users to numerically evaluate derivatives of C/C++ functions for applications such as gradient-based optimization and sensitivity analysis, without needing to derive and implement analytical derivatives.
Type: Cloud-based Test Automation Platform
Founded: 2015
Primary Use: Web, mobile, and API testing
Supported Platforms: Web, iOS, Android, API