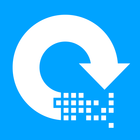
Description: Scan2CAD is a software that converts scanned drawings and images into CAD files. It uses advanced vectorization technology to trace raster images and convert them into editable DWG/DXF files or vector PDFs.
Type: Open Source Test Automation Framework
Founded: 2011
Primary Use: Mobile app testing automation
Supported Platforms: iOS, Android, Windows
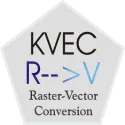
Description: KVEC is an open-source knowledge vector embedding creation toolkit. It allows users to create customized word vector models from text corpora for use in natural language processing tasks.
Type: Cloud-based Test Automation Platform
Founded: 2015
Primary Use: Web, mobile, and API testing
Supported Platforms: Web, iOS, Android, API