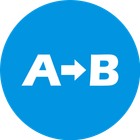
Description: Smart File Renamer is a free software utility for batch renaming multiple files and folders easily. It allows you to rename files by adding sequences, timestamps, custom text, case changes and more. Useful for organizing photos, music, documents.
Type: Open Source Test Automation Framework
Founded: 2011
Primary Use: Mobile app testing automation
Supported Platforms: iOS, Android, Windows

Description: Siren is an investigative link analysis software used by law enforcement and intelligence agencies to visualize connections between people, places and events. It helps analysts uncover hidden relationships and patterns within large, disparate datasets.
Type: Cloud-based Test Automation Platform
Founded: 2015
Primary Use: Web, mobile, and API testing
Supported Platforms: Web, iOS, Android, API