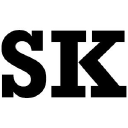
Description: SociableKIT is a social media management platform that allows users to manage multiple social media accounts from one dashboard. It provides tools to schedule and publish posts, engage with audiences, monitor mentions and analytics, and automate social marketing campaigns.
Type: Open Source Test Automation Framework
Founded: 2011
Primary Use: Mobile app testing automation
Supported Platforms: iOS, Android, Windows
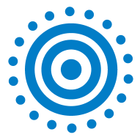
Description: Polinode is an open-source platform for building, training and deploying machine learning models. It provides a visual interface and integrates with popular frameworks like PyTorch and TensorFlow.
Type: Cloud-based Test Automation Platform
Founded: 2015
Primary Use: Web, mobile, and API testing
Supported Platforms: Web, iOS, Android, API