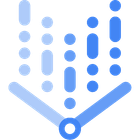
Description: Vertex AI is Google Cloud's managed machine learning platform that allows users to easily build, deploy, and maintain ML models. It provides tools for the full ML lifecycle including datasets, training, serving, monitoring, and more.
Type: Open Source Test Automation Framework
Founded: 2011
Primary Use: Mobile app testing automation
Supported Platforms: iOS, Android, Windows
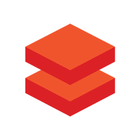
Description: Databricks is a cloud-based big data analytics platform optimized for Apache Spark. It simplifies Apache Spark configuration, deployment, and management to enable faster experiments and model building using big data.
Type: Cloud-based Test Automation Platform
Founded: 2015
Primary Use: Web, mobile, and API testing
Supported Platforms: Web, iOS, Android, API