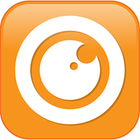
Description: CloudMonix is a cloud management platform that provides visibility and control across multi-cloud environments. It offers features like automated discovery, dependency mapping, cost optimization, security, and compliance.
Type: Open Source Test Automation Framework
Founded: 2011
Primary Use: Mobile app testing automation
Supported Platforms: iOS, Android, Windows
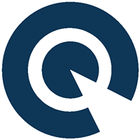
Description: Corlysis is an open-source alternative to Minitab Statistical Software. It is a desktop application for Windows, Mac, and Linux that provides data analysis, statistical modeling, and data visualization capabilities. Corlysis allows importing, manipulating, analyzing, and visualizing data sets.
Type: Cloud-based Test Automation Platform
Founded: 2015
Primary Use: Web, mobile, and API testing
Supported Platforms: Web, iOS, Android, API