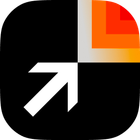
Description: CVAT is an open source computer vision annotation tool for labeling images and video. It allows for collaborative annotation of datasets with features like predefined tags, interpolation of bounding boxes across frames, and review/acceptance workflows.
Type: Open Source Test Automation Framework
Founded: 2011
Primary Use: Mobile app testing automation
Supported Platforms: iOS, Android, Windows
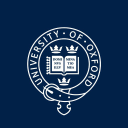
Description: VGG Image Annotator (VIA) is an open source image annotation tool for labeling images to create datasets for machine learning models. It supports region, rectangle, ellipse, polygon, point, and image-level annotations.
Type: Cloud-based Test Automation Platform
Founded: 2015
Primary Use: Web, mobile, and API testing
Supported Platforms: Web, iOS, Android, API