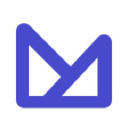
Description: Meltano is an open source data integration platform that makes it easier for data engineers and analysts to connect, transform, and load data. It includes a visual interface for building data pipelines, manages underlying infrastructure, and standardizes workflows.
Type: Open Source Test Automation Framework
Founded: 2011
Primary Use: Mobile app testing automation
Supported Platforms: iOS, Android, Windows
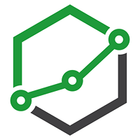
Description: Holistics is an AI-powered unified data platform that enables data teams to build, unify, operationalize, and govern all their data assets for analytics and machine learning. It allows easy data ingestion, preparation, analytics, and visualization while ensuring security, privacy, and governance over data.
Type: Cloud-based Test Automation Platform
Founded: 2015
Primary Use: Web, mobile, and API testing
Supported Platforms: Web, iOS, Android, API