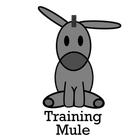
Description: Training Mule is an eLearning software designed for employee onboarding and training. It allows you to create interactive learning content with assessments, mobilize training materials, track learner progress, and more.
Type: Open Source Test Automation Framework
Founded: 2011
Primary Use: Mobile app testing automation
Supported Platforms: iOS, Android, Windows
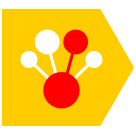
Description: CatBoost is an open-source machine learning algorithm developed by Yandex for gradient boosting on decision trees. It is fast, scalable, and supports a variety of data types including categorical features without one-hot encoding.
Type: Cloud-based Test Automation Platform
Founded: 2015
Primary Use: Web, mobile, and API testing
Supported Platforms: Web, iOS, Android, API