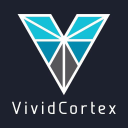
Description: VividCortex is a database monitoring and analytics platform designed specifically for MySQL, PostgreSQL, MongoDB, Redis, and other databases. It provides deep visibility into database workload, queries, performance issues, and trends.
Type: Open Source Test Automation Framework
Founded: 2011
Primary Use: Mobile app testing automation
Supported Platforms: iOS, Android, Windows
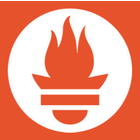
Description: Prometheus is an open-source systems monitoring and alerting toolkit. It collects metrics from configured targets at given intervals, evaluates rule expressions, displays the results, and can trigger alerts if certain conditions are met.
Type: Cloud-based Test Automation Platform
Founded: 2015
Primary Use: Web, mobile, and API testing
Supported Platforms: Web, iOS, Android, API